Explainable Robotics
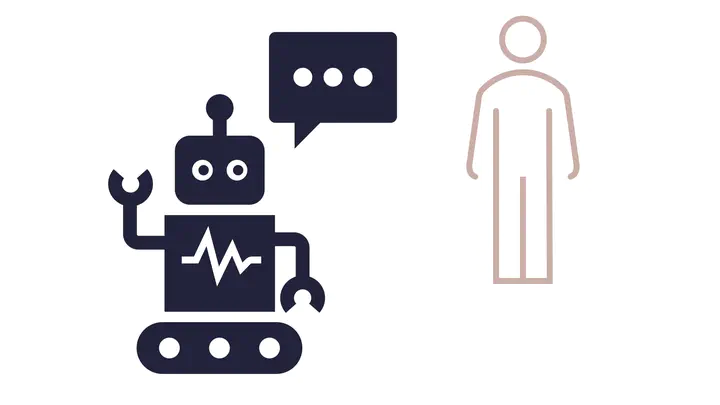
Explainability refers to a robot’s ability to communicate why it acted in a certain way. Such explanations help users understand robot behavior and calibrate their trust appropriately.
Many mechanisms to explain robot behavior are developed in isolation from real collaborative contexts. However, when collaborating with a person, additional questions arise concerning explainability:
- Explanation timing: When do explanations become necessary? Can the robot explain proactively?
- Explanation selection: Given the complex processes underlying robot behavior, how do you select an explanation that answers the user’s question?
To tackle this problem, I conducted studies establishing when users want explanations from a robot. The results reveal a set of scenarios in which the ability to give explanations is key, for example, when the robot makes errors, is uncertain, or is unable to achieve a task. Building on these insights, I developed neurosymbolic agent architectures that leverage foundation models and symbolic planners to provide the right explanation at the right time.
Additionally, I explored the use of non-verbal user cues, such as eye gaze, to detect confusion and its source. Interpreting these signals enables robots to generate user-centered explanations proactively.
This approach ensures that robot explanations are both relevant and timely, enhancing trust calibration and team performance.